Creating reusable knowledge: how to design effective experiments
Innovation teams can generate value in several ways, and creating reusable knowledge is one easy way to do so.
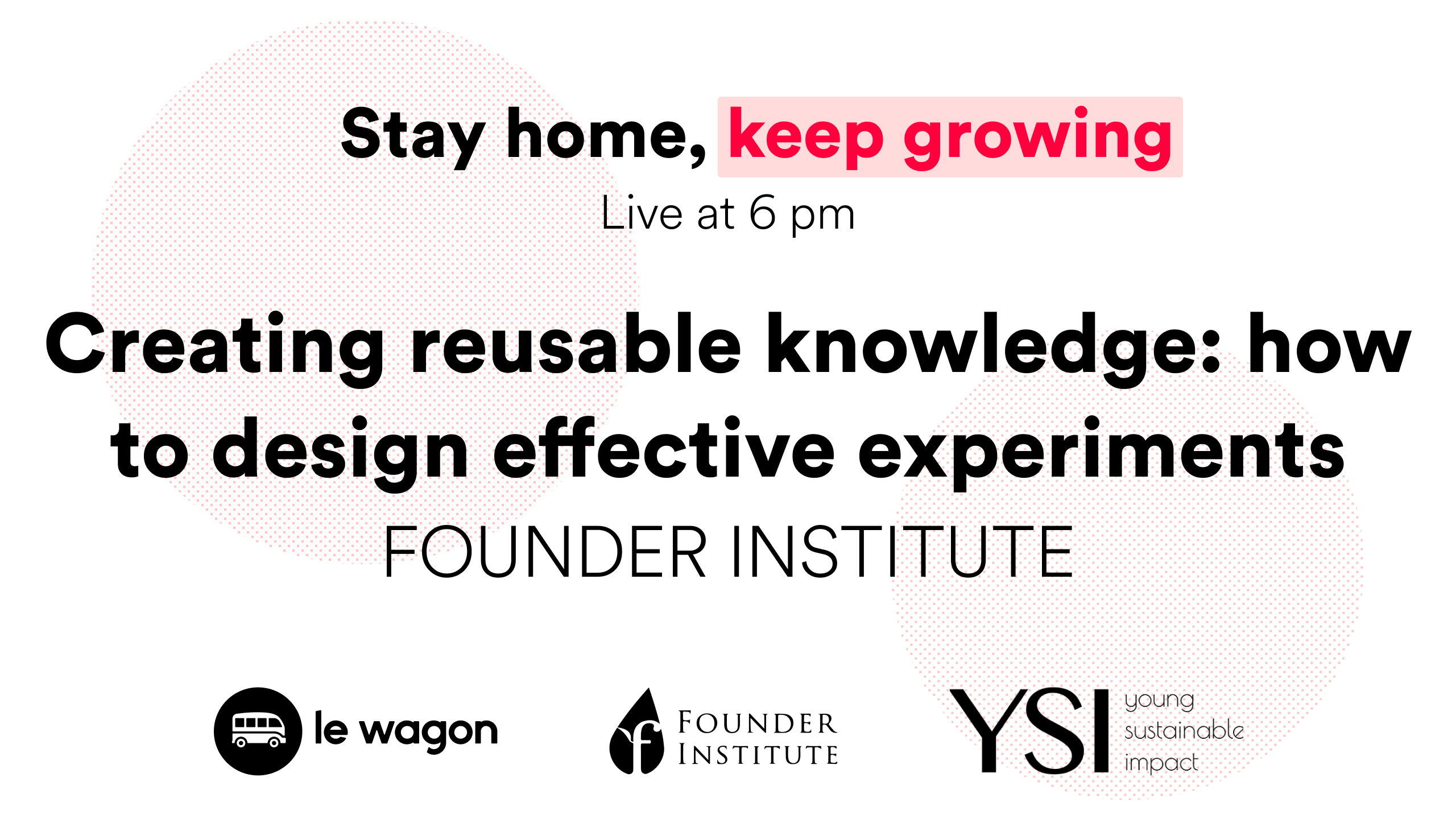
If your innovation teams are not creating reusable knowledge then they are creating waste by (most likely accidentally) discarding knowledge!
"Remember, kids, the only difference between screwing around and science is writing it down!" – is an appropriate quote from Adam Savage that comes to my mind.
One of the five principles of Lean Startup is the Build - Measure - Learn loop. In order to design an effective experiment you have to start backwards! Ask yourself – what is it that you want to learn? How can you measure that? What do you need to build in order to learn that?
Innovation teams can create value in several ways, and creating reusable knowledge is one easy way to do so. Not only does it help the team in question, but also contributes to overall organisational learning which is one of the pillars of long-term competitiveness.
In Creating reusable knowledge: how to design effective experiments webinar, I explain ten steps for designing and learning from Lean Experiments.
You can find recording, timestamps, and transcript below.
License information is included at the end.
This webinar was a part Stay home, keep growing series of online events.
Webinar recording
Download link.
Webinar timestamps
Time | Topic |
---|---|
04:00 | Creating reusable knowledge |
07:28 | Experimentation in three phases |
10:58 | Ten-steps for designing lean experiments |
14:35 | Step 1. Define learning goal |
18:00 | Step 2. Describe who will you learn it from |
21:25 | Step 3. Detail the experiment you will conduct |
23:00 | Step 4. Define fail or success criteria |
27:20 | Step 5. Define time boundary |
29:54 | Step 6. Test the experiment |
31:18 | Step 7. Run the experiment |
32:34 | Step 8. Capture and document results |
33:48 | Step 9. Analyse and interpret the results |
34:36 | Step 10. Decide about next steps |
37:05 | Knowledge creation process |
37:38 | Knowledge capture process |
39:15 | Closing |
Webinar transcript
Hello and welcome to Creating Reusable Knowledge webinar.
My name is Bruno Pešec and I will be your host. Before we jump into the sexy stuff, I want to cover some technical details.
So, those of you joining via Zoom, there is a Q&A box on the bottom of your screen that you use to leave questions. There is also a chat function. A reminder that if you use the chat function, make sure it is to all so that we can see your question.
This event is part of a broader set of events called Stay Home, Keep Growing organised by Founder Institute, Le Wagon and Young Sustainable Impact. The website is very simple; stayhomekeepgrowing.online and you can access all previous events and future events there.
Now, before I jump to the core of the topic, I juts want to give you a short introduction about myself so you can be aware of my biases and where do my comments come from.
So, as I said, my name is Bruno Pešec and I discovered a wonderful world of experimentation 12 years ago while studying for industrial engineering and I had a course called Engineering Statistics. Now that is pretty much hardcore statistics; all applied statistics, especially in the field of quantitative use of data.
So, those were things like designs with mixtures and so kind of, you would have molten steel and more part of each component, so you can get the perfect strength of steel, hardness, malleability or some other characteristics.
For my Bachelors I actually designed an algorithm for the design of experiments using mixtures and then I went on and actually used that knowledge in the industry, working in defence and manufacturing, on battle tanks, weapon systems, freight trains, pressure vessels and so on to calculate some stuff because quantitative experiments are extremely valuable because they bring up real stuff.
It is really expensive and so you want to run as many simulations as possible so that once you decide to blow up something that costs a lot, that you are sure that you are getting exactly what you need.
As I started to move out of the manufacturing industry and into more of the service industry, working with a broader spectrum of problems, I started using qualitative experiments. So, that was more on finding out the meaning and why do things happen and that’s where I also realised that there is some limitation to both of those.
Yes, there is a mixed model of designs, but they also don’t really answer everything and at the core of the problem is ages, thousands of years long debate around what constitutes knowledge, what constitutes truth an how do we get to know and that is the philosophical debate that I will not go into, but I will share with you today is base don my ten years of experience of getting real results in real settings, as practical as possible as I made it to be after so many years of practising.
Now, this is quite a challenging topic and so I suggest that you have a pen and a paper and write your own notes and ask questions as we go. If you will have a question later, which is quite possible, just send me out an e-mail or find whatever contact point and ask your question. It costs nothing. I will get back to you when I can.
Now, let’s start.
I call this one Creating Reusable Knowledge and the subtitle is about effective experiments and the important thing here is the core issue.
Why do you want to do experiments in the first place? Yes, it is a fun activity for freaks like me, but in general, it is not really a sexy activity, especially when you start putting in analytics, critical thinking, validity. Oh my god, when you go into validity constructs, it goes really bonkers.
So, it is like, why do experiments in the first place?
Because you want to make a decision; a better decision. That is one and the second one is because you want to create value; both for the customers and for the organisation that you are part of. It doesn’t matter if you are an entrepreneur or if you are in a massive organisation. You need to create both organisational value and customer value.
Now, for something to be reusable, I mean, bad stuff can be reusable as well, but I hope that you don’t want that. The point is creating trustworthy knowledge; something that others can trust and that you can trust t use again and again and again.
Now, this is a really, really trivial example of reusable knowledge. When I went to elementary school in Croatia, what was really popular for mathematics were small tables. There were like multiplication tables and that is a form of reusable knowledge. You have a multiplication table, you have it right in front of you and without calculating or without thinking, just read out the result and you can trust that result because well, as a kid, you trust it because your parents and teachers say you can trust it, but as an adult you trust it because you know that 2x2 is 4. 4x4 is 16 in a decimal system, at least. So, that is an extremely trivial example.
We can create such reusable knowledge.
It has multiple benefits and so within the same team it is for making better decisions, but as teams start to grow and you start to bring more people on, it is about onboarding them.
An organisation has multiple teams, multiple sections, multiple divisions and it is about multiplying the value of work because if one team spent one week experimenting with something and they did in such a way that every other team can trust it and reuse it, you saved a lot of time and if those teams actually can take that knowledge and create or improve their products or services; operations or whatever, you have created value out of thin air and that is magic and that is why to me, I am so obsessed with experimentation.
Here, when I use the experimentation it is not purely in the meaning of the scientific method where we have independent variables and we are tweaking them and seeing what the response is, but it is broader meaning of the word, experimentation as figuring things out in a structured and systematic way, with a purpose of learning something new to make a better decision.
That is how I view it and now when we have framing, let’s go into it.
So, prepare for a crazy journey. At the very high level, experimentation has always three phases; they are not symmetric, but that’s okay. We will go into that detail.
So, first phase is, design the experiment. The second is conduct the experiment and third, learn from the experiment.
Now, it is very important to give proper attention to every phase because you don’t want to get CICO – crap in, crap out. You don’t want that. It’s a waste of your time.
How you can do each, we will go through in detail in minutes to come, but first to visualise this as a step by step process. I found that it’s quite valuable to visualise that process, especially if you struggle with intangibles and if you work with someone who is more of a practical oriented person who doesn’t work very well with abstract or whatever. Kind of drawing that up and showing the steps helps a lot before we start explaining them.
The three phases can also be visualised like this. You basically start with assumptions. Now, how are assumptions framed? It is basically subconsciously and they are as a result of the environment, of our upbringing, of what we are curious about, of what we are focused on, about everything that is happening around us and what is guiding the organisation. Assumptions are formed all the time.
What is important is when working on innovative ideas or on something that you want to learn more about, is becoming aware of the assumptions. There are several tools, several methods or models you can use like assumption mapping, hypothesis prioritisation matrix etc. They are all tools to surface assumptions specifically within the start-up, product development, product management, and agile context.
The reason for surfacing them is because you want to translate them into hypotheses that can be tested. I will come back a bit later on the difference between assumptions and hypotheses. These hypotheses are then tested and experimented upon and you extract learning from that.
Simple. Trivial. Right?
Let’s remind you of the three phases. Just looking at this figure, think for yourself for five seconds where do these three phases manifest? In which of these four steps?
Let’s see where phase one is. Yes, phase one is all three of these, including experiment. So, designing an experiment involves stating the learning goal, stating the hypotheses and then building the whole experiment around it.
What will you measure? How will you measure? How will you explore it? So, all of this is here. The phase two, conducting the experiment is basically running the experiment that you designed and the last phase of learning happens here. Now, we will go into details of ten steps.
So, those three phases I divided into ten generic steps that you can use absolutely every time to design a lean experiment and here, I use lean experiment as I have described a moment ago.
So, step number one is, start with the learning goal.
Step number two is define who will you learn it from.
Step number three is detail the experiment and step number four is, define fail/success criteria. Very important.
Step number 5, define time boundary.
Step number 6, test the experiment itself. You don’t want to fail for no good reason.
Step number 7, run the actual experiment.
Step number 8, capture the results.
Step number 9, interpret them; what everybody has been waiting for.
Step number 10, make the decision.
Remember what I started with? You want to run an experiment because you want to make a better decision. Not for shit and giggles. I mean, you can, but nobody is going to pay you for that. Or if they are, give me a call. Maybe they pay me as well.
Okay, back to the three phases.
So, where do you think that these three phases manifest, visually, in these ten steps?
Again, I will give you ten seconds to think and then I will show you.
So, design is first five steps. You can see, I deliberately visualised it like this so you can see how much love and effort has to go into design of the experiment and it pays off. It is extremely important. Running experiments is trivial.
Making dumb ass conclusions is easy. Anyone can do it. Actually, learning from something you can trust takes effort, skill, knowledge and dedication and that is what we are going to go through.
Now, conducting are these two boxes and I am including a test experiment, based on my experience with a lot of teams. Sometimes it happens that perfectly designed experiments failed because of the wrong button that was pushed.
Finally, learning is divided into three stages because we don’t have perfect brains. We are humans and that is perfectly fine, but we have to work with it. It is our responsibility to work with that in order to get the most trustworthy data.
Now, I will walk you through each step and give you the most important pointers. It is impossible to transfer whole breadth and everything that needs to bedone in each within 45 minutes, but I will give you enough to work with and I will give you plenty of leads that you can explore further and I have some resources I can follow up with, so don’t stress.
I still invite you to keep writing on your piece of paper or notebook because this is a lot of stuff. It can be quite overwhelming and it will help you later to revisit your own notes and ask better questions so others can help you, better.
So, let’s start with step number 1.
So overlooked. It is what I always ask when I am working with a team. What is it that you want to learn? Every great experiment starts with a clear learning goal.
If you don’t know what you want to learn, then you can do whatever and you can see that sometimes. Sometimes we are so clueless that we can start anywhere, but then you are not looking at experimentation.
You are looking at some other type of work that you should do before you actually start experimenting and that’s perfectly fine, but if you are trying to experiment as being totally clueless and not even knowing what you want to learn, that’s a recipe for failure. That is a recipe for disaster.
So, defining what your learning goal is, is an important step. It is what kicks everything off. That can be a broad statement. It can be something we want to learn if people will buy this.
We want to learn if people will like this. That’s an acceptable starting learning goal; start a learning goal. Now, you want to go more in detail and be more specific. So here, we have the question of maturity, of context and now, when you have learning goals, what do you want to translate it to or what do you want to derive from it are the assumptions I was talking about.
What are you assuming? If you have the learning goal, that’s we want to find out if people will like that. Okay, so what are the assumptions you want to focus on? Are you making assumptions on the type of people or on what will they like, how will they like it, what will they appreciate?
Then, you want to translate, if possible, these assumptions into hypotheses and so the difference between an assumption and a hypothesis is how are they stated.
Assumptions are broad and unspecific. That is perfectly fine. Hypotheses are narrow and specific.
So, while assumptions can be, we think or we believe that these people will like something. A hypothesis would be, this specific thing in this specific context, with these specific people will create these specific results. That’s one form of a hypothesis.
Assumptions can be researched and hypotheses can be tested.
That is the core difference and that is something that should guide you all the time. If you cannot translate the assumption into hypotheses, that means one of two things.
One, you actually don’t know enough about the learning goal. You don’t know about the context and you need to do more research. That’s not a problem. Then you switch to running research, you write down research questions and relate them to qualitative experimentation or second, you actually lack skills in writing hypotheses. Then go online, read.
When you have these two, the next step is, who are you going to learn this from?
Experimentation doesn’t happen in a vacuum and since we are talking about lean experimentation you are most likely to want to experiment something related to business. You will not do Petri dish and you will not be blowing up battle tanks. For that, there are traditional designs of experiments. So, the things that you want to learn, you must learn from real humans and you must be specific about who you want to learn it from.
Traditional descriptors are demographic data and psychographic data. For start-up’s or for corporate teams that cannot get funding, that is sometimes difficult to get.
For start-up’s, you can go into different databases and try to dig. For corporate teams,you can go to your marketing department and ask for that. Sometimes you can get it and sometime you don’t, but do not despair. That is not the most important descriptor within lean experimentation.
In fact, it is actually three others that are more valuable and that can get you further, faster.
So, whenever you are describing who want to learn from, one of these three should be present. What is the problem that these people are having or what is the undeserved need they have or what is the job they are trying to get done?
It is one of these three that you should focus on that should always be present.
Another thing about this step, who are we going to learn it from is something that comes from statistic sampling.
Sampling is very, very important. Trustworthiness is all about sampling. If you go on the street and speak with the first five people you meet, that is not a random sample. You might think it is a random sample, but it is an extremely biased sample of people who might not fit who you want to learn from.
If you are working on a financial product for bankers and you go and have interviews with people on the street and you speak with ten people. How many of them do you think are going to be the target customer segment that you want to learn from? Probably not very many.
So, it is important to be specific on who you want to learn it from and when it comes to sampling, random sampling is always best when you can. If you have got a list of people and you pick the first one; that is not a random sample, again. That is just the first time. It is a biased sample.
How you do sampling has direct correlation with the trustworthiness and validity of your data. In the beginning, sample sizes can be small, but the more mature the idea is, the more difficult the experiment is. Sample sizes need to be bigger and bigger and bigger. If you are doing quantitative experiments, they need to be quite big. That is 500 or more. If you are doing qualitative it is okay if they are small.
It is actually rule of thumb for qualitative experiments, five is good enough if and only if all five have been pulled at random, all five are from the sample that you described and all five are for the same learning goal. Then, that is perfectly fine. It is not something that you should decide to invest 100 000 000 Euros based on, but it is perfectly fine to get you to the next step.
Now let’s go to step number 3.
Once you have assigned the learning goal, the assumption, hypothesis and who you want to learn from, then you finally go, how are you going to learn it?
Here, there is a lot of cook books. What became really popular is the start-up style of experimentation. So that is customer interviews, MVP’s and so on; landing pages and what not.
So, what I suggest for beginners and teams of the like, is it is so easy once you know what you want to learn and who you are going to learn it from and then just look for experimental designs that fit what you want to learn.
If you know that you are going for qualitative or quantitative, then it can be easier. Then we are looking at what are we actually capable of? What do we have access to? What are we allowed to execute?
So, corporate teams cannot do any experiments. Smoke tests where you are kind of pretending you have an offer, but people say yes, I want it and you say, “Oh, we actually were faking. We don’t have that offer.” You know, not all corporates are allowed to do that.
So, it is your responsibility to pick an experiment or design that fits and is allowed within the organisation. This step is all about how are you actually going to learn it and it is so much easier to answer that, as I said, if you did proper steps for one and two, but even when you describe how you want to learn it, that’s not enough.
You still cannot just run out and do it. You must do two more things.
You must define a fail or a success criterion. These are essentially the same thing, but just decide from which side to define them and the reason I included the same or two words is because sometimes, within large organisations and corporates, words matter. Some people just have strong distaste for failure. Failure doesn’t exist in this organisation. You then talk about success criteria and flip it.
There are three ways to set fail or success criteria and I will go from topto bottom, based on how strong they are recommended. So, the first one: the best one is if you can derive it from the actual business case and that can come from profit formula, revenue streams; whatever is assumed there or whatever the model is, or example, if you have access to resources doing Monte Carlo simulation; that is a bit overkill. That is for more mature ideas, but you can see some potential outcomes. A lighter way is doing TAM-SAM-SOM analysis and that is a form of marketing analysis and then calculating from that kind of what you believe to be realistic and then dividing that with a little bit more or you are usually a bit more optimistic. Then go from there. That is number one: derive from the business case.
Number two is the industry standard. So usually whatever you are trying to learn, there is some number out there already. It could be in an industry-specific report, it could be from competitor analysis, but in most cases, you will be able to find it simply by Googling. If you cannot Google, go to your local university library and ask if you can access the archives or the databases because sometimes university libraries have, even for visitors, free access to different economic databases. That is a really good source of information.
The third one is Hippocratic oath or do no evil. So, you most likely won’t be doing something in a vacuum and you have probably done something similar or someone from your organisation or actually did something similar and then you can look into, “Okay, we don’t want” or, “We will not accept the option that is under performing our current level of performance.” Very simple.
So, these three you can always pick.
There is a fourth one, which is a fall-back if you cannot define it in any of the first three ways and as I said, it is a fall-back. I caution against it, but I will share it.
So, the fall-back is, what is the smallest result that will justify me spending more time on this?
Why I say it is a fall-back and caution against it, especially if you are alone or if you have a very strong personality and you are leading a team, it is quite easy to get delusional.
So, if you are using that as a fall-back, make sure that you have a trusted advisor or a trustworthy sparring partner who can openly discuss it with you and give you feedback if he/she sees that you are kind of putting something so small, that basically they don’t know why you are doing that, then just don’t do an experiment. Just go create, code, deliver, deploy and just go an do it. Don’t pretend to do experimentation because then you are just wasting time.
Those are three ways to define fail or success criteria and you should define them before running the experiment to defend yourself against the hindsight bias.
So, what you don’t want to do is when you get the results and then talk, “Is this acceptable to us or not?” Let us move this goal post Suddenly it is acceptable. You want to define that in advance. Then, when you come to step 9, you can have a really candid discussion with yourself or with your team or both about what do these results mean.
One more thing before we run the result or experiment is, you should define the time boundary.
Time boundary is something to help you with the speed.
Usually my recommendation is for entrepreneurs, go for week. Challenge yourself.
If in step 3, I designed an experiment that I don’t think I can actually do in 1 week, but it will actually take me 3 weeks; then I challenge you back. Can you rethink this in 20 minutes; the same learning goal, the same assumption, the same hypotheses, the same people you want to learn from, but different experiments, different ways to learn it in less time and with the less money. Go back, 20 minutes that can save you 1 week. Isn’t that a great deal?
In the corporate setting. It is a bit more difficult, especially if it is B2B because sometimes cycles are a bit longer, it can be difficult to get especially if you are going to key account managers or if there is some sort of bottleneck or gate keeping function, it might be difficult to get to people.
There are two ways to handle that. One is that you can say, “It will be a week, but it will start once we have arranged all the meetings.” Or second, you can go back to step three and rethink if there are different ways to learn this.
Alternative to time boundary or something that can be used in conjunction with time boundary is if you have defined in step two, the sample size you can say, “When we have reached the desired sample size, we will stop.” So, it can be, for example, two weeks or we have reached 400 people. Both are perfectly fine.
Sometimes we can go really fast. I had that happen many times, especially if you are doing quantitative experiments that are in digital channels. They are ever so popular. So, if I need two groups of 1000 people and I reach that within 2 days, stop it and let’s move on. Let’s move to step 8, 9 and 10 because there is no point in moving on. I am learning a specific thing. That’s what I want to learn from and then I will continue. I would rather do one more experiment than extend the same experiment if I know the sample size that I want.
Now, when you have a rock-solid design. So going into these 5 steps, you have a rock-solid design that you can argue and you can use and reuse and come back to and study and interpret and everything.
Now, you can finally go into conducting the experiment, but before running full force on your whole sample size, it is very smart to test it and here, testing is not to get an early dip into the results or the outcomes, but it is testing the tiny technical stuff of your experiment.
So, if you have decided to do some customer interviews, make sure that the questions make sense. Just grab someone. You don’t need the actual sample to make sense out of the questions. So, make sure that the questions make sense.
If you decided to do something in digital channels, make sure that all links work, make sure that you are tracking these if you are usingGoogle analytics or using Kiss Metrics or whatever you might be using. Make sure that everything is connected properly.
If you are not in control of sending out the experiment to the right people, make sure that they do their job properly. So, I had a team that did the first five steps perfectly and they prepared everything. Everything went out, the results came back and they made no sense at all. “Wow, we did not expect this at all.” Then, when we were looking through that, it was like, “Oh the experiment went to the completely wrong people.” The person decided that since they can’t the sample size, they just expanded it and it was completely irrelevant for that learning goal.
After we have tested it, then just go.
Now, depending o the step 3, which experiment you designed, how you want to learn it, you might be very actively involved in interviews and anything similar to that or it may be very passive. You design an experiment. It is in the digital channels. Ads are running, testing is happening and something else is calculating. You just chill.
What is important in that period is to resist the urge to take sneak peaks. Resist that. Go do some other productive work. Plan other experiments. Think about what you will do with this one; whatever. Just go and chill. You will bias yourself unnecessarily. You might by accident start tweaking variables and then you will not get the trustworthy stuff.
The only excuse to stop it is if someone is getting hurt and that can mean your brand, your organisation or people that are being deployed to. That is a reason to immediately stop it. I had cases like that when suddenly the phone rings and competition calls, “Oh we have seen that. What is that?” That is a valid reason to pull the plug.
Now, you have done the experiment and step number 8 is dumb as hell.
Very important. You need to capture the results. That is not interpreting the results. You need to write them down how they are. If you are doing quantitative experimentation it can be 78 out of 111 said yes or whatever it was. 11 out of 18 walked out. 28 out of 55 did that or this in that specific setting.
If it was qualitative, capture observations and not interpretations. Here is the difference. He is angry. That is an interpretation. He crossed his hands and he raised his voice. That is an observation.
You want to have a list of observations and numerical results. You want to capture that so you have that separate from the interpretation that comes later. Very, very important because when you are becoming back or when you try to reuse that reusable knowledge if you have merged that, it will be very, very difficult because sometimes our interpretations can be questioned, but numbers can rarely be questioned if they were recorded properly.
Step number 9, interpretation.
That is what we are living for, finally. That is the prelude to actually making a decision. That is making sense of the data.
So, everything that we have written down; qualitative, quantitative; everything that you did in the steps 1-5 and steps 6-7 and now you are making sense out of it. You have background, you have context. Write it. What do you think that means? How is that reflected? What do you do based on that? What implications does it have to you to the rest of the teams or other assumptions you have or in the future what you want to do; everything goes here.
Go wild. Write as much as you want and then summarize it.
That should support directly the decision you are proposing in step 10.
So, there are basically three decisions that you can make. You can continue with the work, you can stop with the work or you can pivot. Pivot; changing strategy without changing vision. You can do any three of those.
What you decide to do should be directly supported on what your insight is from step 9.
So, when someone comes to me and says, “Well, this is what we decided” and I say, “Show me what lead you to this decision.” That is what I am going to look for. I will work my way backwards. “Okay, there’s a decision. That is the thinking behind it. Those are the numbers behind it. How did you get those numbers? Where did they come from? Who did they come from?” Working backwards.
When you finish an experiment and you write it down and you made a decision, even if you have acted upon it, check it backwards.
So, each should make sense, both ways. 1-10 and from 10-1; that is an inexpensive way to check, especially validity without going into philosophical discussions about different forms of validity, validity constructs and how they work.
These are the ten steps in a very short amount of time. Everything you need to know about these ten steps and if you follow them, I guarantee you that you will get trustworthy learning that you can turn into reusable knowledge with as little work as possible.
Before we wrap up, some more things. These ten steps as we went through them, I invited you to prepare some questions. I will check them now. If there are none, I will start wrapping this up and say my nice thanks. Let me just open this. There’s a message.
So, this has been either so clear or so overwhelming that there are no questions. I did talk about everything and I am sharing this guide. So, this is the short link to it. If you go there, you will find all the ten steps described in detail together with links to all resources, including advanced resources at the bottom of the text.
To sum this up, I want to kind of go back to the beginning.
Why do we want to run experiments?
We want to create reusable knowledge and the process to do that is very simple.
You start with your environment and what your learning goal is. You make assumptions based on that. Assumptions are in this contest for the success of the business. We try to translate them into testable and falsifiable hypotheses which then in turn you try to figure out, how can I learn this and more about it?
That is experimentation.
You conduct it and you learn it and then the documentation or capture process, as simple and as trivial as it is, is critical. So, you need to separate the result from the interpretation. Write down both and be clear which is which.
That is what creates value long-term. Short-term it is about making a decision and long term you are accumulating this amazing reusable knowledge, which is a source of genuine competitive advantage.
Let’s look at Toyota and Toyota Production System. That is one of the best, if not the best production system in the world since the ‘50’s. There are so many books. My bookshelf is filled with Toyota books, Lean thinking, Lean manufacturing, Lean production and yet, there is almost no companies in the world that came even close to them because they repeatedly; they are the masters of creating reusable knowledge, repeatedly.
It is built at every level of the organisation and it is something that even when you see it and they tell you, “This is what we do.” You cannot just walk in and copy. Brains are not copyable and that you can also use to leverage no matter how small you are, no mater how big you are, you can always have better processes for creating and capturing knowledge and that is something tat truly pays off and that you can always argue for financially, organisationally and from a customer perspective.
Now, as I said, due to the format of the webinar, it is great to hear and listen. If you would like a presentation or if you would like any additional materials just hit me up on this e-mail: bruno@pesec.no for this recording and for the recording of other events in the Stay Home, Keep Growing; they are all available on the website stayhomekeepgrowing.online.
There have been many different topics and I suggest you go there and check them out. There have been on start-up process, innovation, well-being, product development, there was coding as well.
Go and educate yourself and become someone better and more successful.
I thank you for joining me tonight and I wish you a success with your business ventures and I wish that you will learn a lot and have a really fulfilling life.
That is it. Thank you very much.
Licences
Webinar recording
Creating reusable knowledge: how to design effective experiments (webinar recording) by Bruno Pešec is licensed under a Creative Commons Attribution-NoDerivatives 4.0 International License.
Based on a work at https://www.pesec.no/creating-reusable-knowledge-how-to-design-effective-experiments/.
Webinar transcript
Creating reusable knowledge: how to design effective experiments (webinar transcript) by Bruno Pešec is licensed under a Creative Commons Attribution 4.0 International License.
Based on a work at https://www.pesec.no/creating-reusable-knowledge-how-to-design-effective-experiments/.
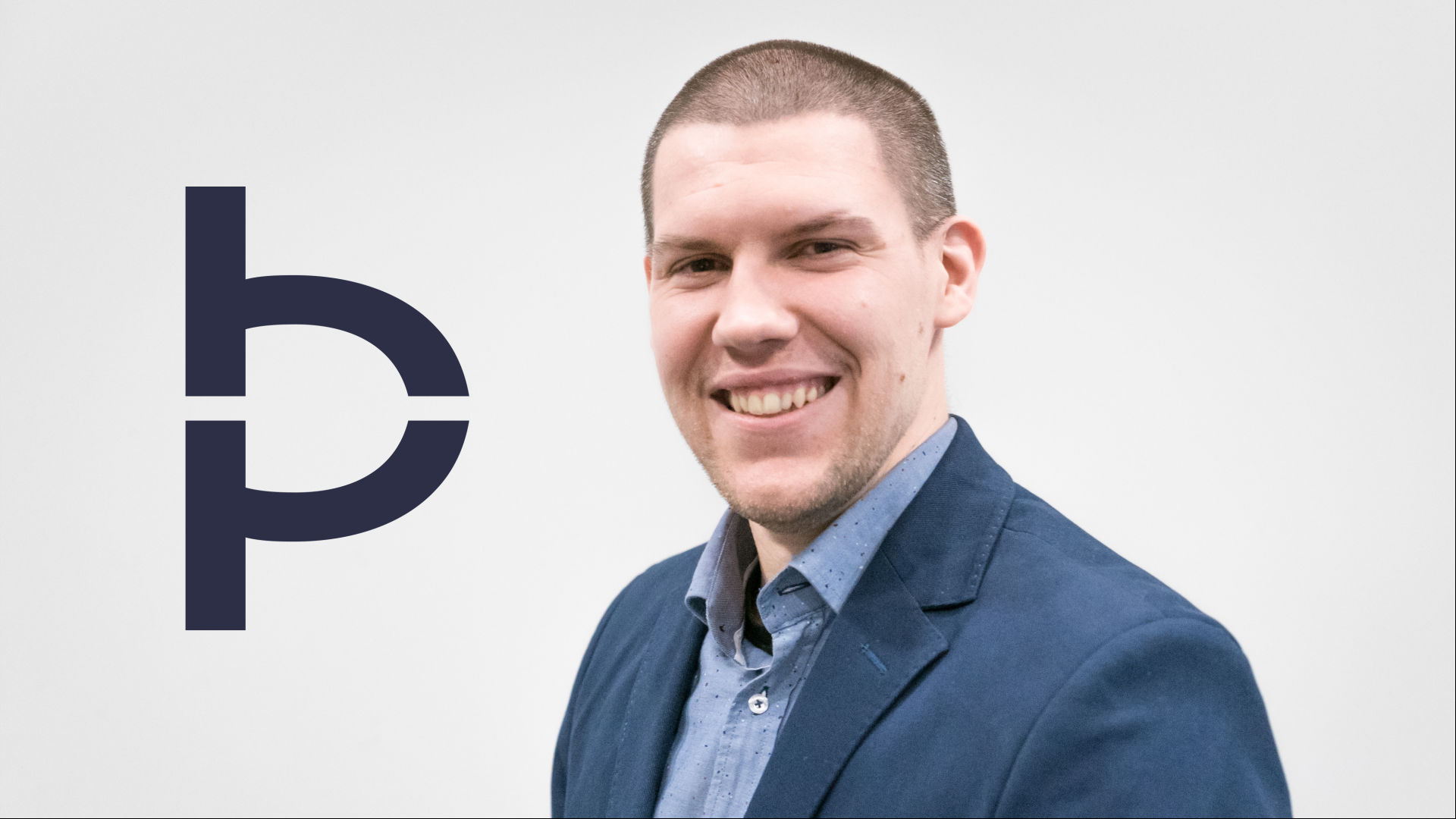
Bruno Unfiltered
Subscribe to get the latest posts delivered right to your inbox. No spam. Only Bruno.