False negatives and false positives
False negative is a result that appears negative when it should not, while false positive is a result that appears positive when it should not.
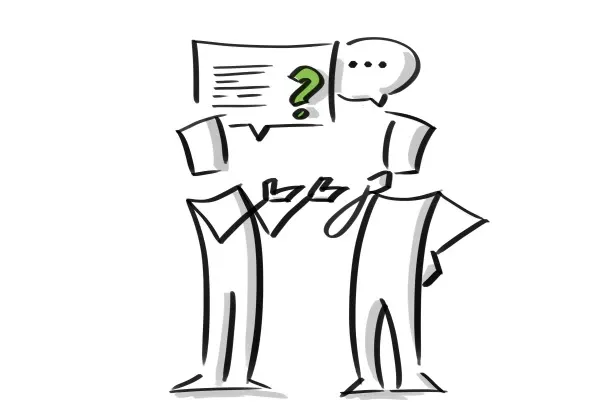
False negative and false positive are two terms that can cause a lot of discussions and misunderstandings. To put is as simple as possible, a false negative is a result that appears negative when it should not. On the other hand, a false positive is a result that appears positive when it should not.
Playing Lean Facilitator Guide, written by Ash Maurya, defines them as:
When your experiment tells you that your idea wasn’t any good, when in reality it would work well, you’re dealing with a false negative. If, on the other hand, your experiment tells you that your idea was good, while in reality it would not work very well, you’re dealing with a false positive. These may come from a flawed test design, biased selection of test subjects or just plain bad luck. No matter the reason, they may lead your team astray.
Don't worry if you're still a bit unsure about these terms. Later in this post we'll discuss where they come from and provide several examples.
Background
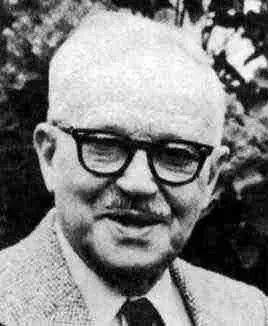
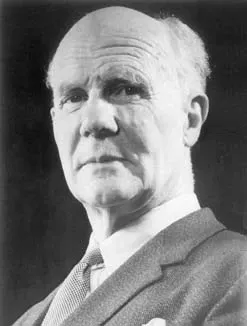
Terms false negative and false positive have their roots in medical statistics. They are related to, but non-synonymous with, the type I and type II error in statistical hypothesis testing. In 1928., statisticians Jerzy Neyman and Egon Pearson identified two sources of error:
- the error of rejecting a hypothesis that should have been accepted (type I error), and
- the error of accepting a hypothesis that should have been rejected (type II error).
As Stats Cat explains it:
If you make a Type I error, you reject the null hypothesis when, in fact, it's true. In our example, the company would conclude that the two cat food formulations taste different when they really don't. Since the cat foods taste the same, this error is not a complete disaster, because at least the cats will experience the same great Puma Gourmet taste regardless of which formulation they get.
If you commit a Type II error, you do not reject the null hypothesis even though it is false. In this case, the company would conclude that the cat foods taste the same when, in fact, they taste different. Imagine how devastating this error would be if, as a result, cats got a less delicious version of their Puma Gourmet food.
If you wish to dig deeper into it, I'd recommend starting with these two well written Wikipedia articles: statistical hypothesis testing and type I and type II errors.
Examples

In her blog post at The Hype Innovation Blog, Oana-Maria Pop provides several examples of false positives and false negatives:
In the innovation manager’s world, a false positive could be a detergent-less laundry system that allegedly cleans laundry by injecting ozone into the cold wash water. From an R&D standpoint, the solution looks ingenious (as ozone kills bacteria 3,000 times faster than bleach), while from a marketing view, it looks like a sure win: after all, wouldn’t we wish away expensive and sometimes harmful washing powder and high electricity bills if we could? In practice though this too-good-to-be-true machine proved to be exactly that: wishful thinking. As independent tests later showed, the ozone did little to remove dirt, blood, grass and other common stains rendering the appliance overpriced and useless. Another example of a false positive is none other than Pepsi’s mishap with Crystal Pepsi. Despite thorough marketing research pointing to customers’ pursuit of purity in all areas of their lives (hence the “clear soda” concept), the beverage failed miserably – not once but twice.
By contrast, a false negative could be a technology or compound that did not seem promising for the core business and was consequently discarded or spun off to seek glory outside the organization. It seems appropriate to mention the classic example of XEROX PARC (Palo Alto Research Centre), as illustrated in H. Chesbrough’s 2002 article Graceful exits and missed opportunities. As the history books show, XEROX spin-offs such as 3Com, VLSI, Adobe, and Komag reported sales figures that exceeded $100 million within seven years of start-up prompting management to reflect on the impossibility to integrate all valuable R&D output into the core business strategy.
Relation to the Lean Canvas

False negatives and false positives can appear during problem and solution interviews, which you have to conduct in order to complete Problem and Solution boxes.
It is important for your success to understand the difference between false negative and false positive, and to be aware of them. Failure to do so may lead you, your team, or your company on a wrong path, with possibly irreparable repercussions.
Example image source: Ellis, P.D. (2010), “Effect Size FAQs” website www.effectsizefaq.com, accessed on 18.06.2016.
This post was originally published on Playing Lean blog.
Bruno Unfiltered
Subscribe to get the latest posts delivered right to your inbox. No spam. Only Bruno.